Getting Started
In this section, we’ll run some “Hello world” primrose
jobs. However, rather than print a string, we’ll do some more useful tasks:
run a read-write job (i.e., read data from a source and write it to some sink; that is, an
Extract-Transfer-Load
job)run machine learning models, including clustering and classification
Your first primrose job
Once you have primrose
installed, you can now run a job. First you’ll need to create your own project directory, by
using the primrose
command line as follows:
primrose create-project --name <myprojectname>
This will create a directory with everything you need to get started, including example config files. Just navigate into this directory to begin.
A read write job
Open config/hello_world_read_write.json
:
{
"implementation_config": {
"reader_config": {
"read_data": {
"class": "CsvReader",
"filename": "data/tennis.csv",
"destinations": [
"write_output"
]
}
},
"writer_config": {
"write_output": {
"class": "CsvWriter",
"key": "data",
"dir": "cache",
"filename": "tennis_output.csv"
}
}
}
}
There are two tasks:
read_data
: this run an instance ofCsvReader
which loads data fromdata/tennis.csv
into a pandas dataframe and sends the data to the next task:write_output
.write_output
: this receives the data (associated with a keydata
) and uses an instance ofCsvWriter
to write the data out tocache/tennis_output.csv
.
It might not be obvious that it is pandas under the hood but otherwise this configuration file should be relatively intuitive.
Running the job
To run the job, type
primrose run --config config/hello_world_read_write.json
You should see something similar to:
2019-07-23 21:15:09,075 INFO configuration.py __init__: Loading config file at config/hello_world_read_write.json
2019-07-23 21:15:09,081 INFO configuration.py check_sections: OK: all sections are supported operations
2019-07-23 21:15:09,081 INFO configuration.py check_config: OK: all class keys are present
2019-07-23 21:15:09,081 INFO configuration.py check_config: OK: all classes recognized
2019-07-23 21:15:09,081 INFO configuration.py check_config: OK: good necessary_configs
2019-07-23 21:15:09,082 INFO configuration_dag.py create_dag: Checking configuration DAG
2019-07-23 21:15:09,082 INFO configuration_dag.py create_dag: OK: good referential integrity
2019-07-23 21:15:09,082 INFO configuration_dag.py check_connected_components: OK: 1 connected component
2019-07-23 21:15:09,082 INFO configuration_dag.py check_for_cycles: OK: no cycles found
2019-07-23 21:15:09,082 INFO dag_runner.py __init__: Traverser is of class <class 'primrose.dag.config_layer_traverser.ConfigLayerTraverser'>
2019-07-23 21:15:09,083 INFO dag_runner.py filter_sequence: Taking nodes to run from default
2019-07-23 21:15:09,083 INFO dag_runner.py run: received node read_data of type reader_config and class CsvReader
2019-07-23 21:15:09,083 INFO csv_reader.py run: Reading data/tennis.csv from CSV
2019-07-23 21:15:09,109 INFO dag_runner.py run: received node write_output of type writer_config and class CsvWriter
2019-07-23 21:15:09,109 INFO csv_writer.py run: Saving data data to cache/tennis_output.csv
2019-07-23 21:15:09,115 INFO dag_runner.py run: All done. Bye bye!
That’s it. You have run a primrose job.
Examining the output
The first half of this output is a set of validation checks against the configuration. You can read more about these checks in DAG Configurations.
The Traverser
is some code that determines the order in which the primrose
nodes are processed.
Importantly, there are four output lines that show the actual work being done:
2019-07-23 21:15:09,083 INFO dag_runner.py run: received node read_data of type reader_config and class CsvReader
2019-07-23 21:15:09,083 INFO csv_reader.py run: Reading data/tennis.csv from CSV
2019-07-23 21:15:09,109 INFO dag_runner.py run: received node write_output of type writer_config and class CsvWriter
2019-07-23 21:15:09,109 INFO csv_writer.py run: Saving data data to cache/tennis_output.csv
You should now have a file cache/tennis_output.csv
that matches the input file data/tennis.csv
.
A Machine Learning Job: Clustering
Now, let’s do some machine learning. We’ll run a basic K-means clustering and plot the clusters.
Plotting the Graph
Before we examine the configuration file, let’s plot a graph of the DAG by typing:
primrose plot --config config/hello_world_cluster_simple_train.json --outfile graph.png
This will write an image of the DAG to graph.png
, without actually running the nodes.
Here we see a read_data
node feeding into a normalize_data
node feeding into a cluster_model
node. The output feeds to write_data
and also to cluster_plotter
.
Examining the configuration
With that background, let’s examine the configuration file. This is `config/hello_world_cluster_simple_train.json
{
"metadata": {
"explanation": ["this is a minimal Sklearn clustering example in training mode:",
" * read in some data from CSV",
" * sklearn.preprocessing.StandardScaler transform the data but no train test split!",
" * cluster with sklearn.cluster.KMeans",
" * predict on same training data, appending predictions column to data",
" * save enriched data to CSV",
" * plot clusters and save image to disk"]
},
"implementation_config": {
"reader_config": {
"read_data": {
"class": "CsvReader",
"filename": "data/unclustered.csv",
"destinations": [
"normalize_data"
]
}
},
"pipeline_config": {
"normalize_data": {
"class": "SklearnPreprocessingPipeline",
"operations": [
{"class":"preprocessing.StandardScaler", "columns": ["x1", "x2"], "args": {"with_mean": true, "with_std": true}}
],
"is_training": true,
"destinations": [
"cluster_model"
]
}
},
"model_config": {
"cluster_model":{
"class": "SklearnClusterModel",
"mode": "train",
"X": ["x1","x2"],
"model": {"class": "cluster.KMeans", "args": {"n_clusters": 6, "random_state": 42}},
"destinations": [
"write_data",
"cluster_plotter"
]
}
},
"writer_config": {
"write_data": {
"class": "CsvWriter",
"key": "data",
"dir": "cache",
"filename": "clustered_output.csv"
}
},
"dataviz_config": {
"cluster_plotter": {
"class": "ClusterPlotter",
"id_col": "cluster_id",
"filename": "clusters.png",
"title": "Results of KMeans(k=6)"
}
}
}
}
Most of it should be relatively intuitive but let’s walk through it.
read_data
uses aCsvReader
to read in some data. This fees tonormalize_data
.normalize_data
is a pipeline, a preprocessing step. This is going to runsklearn.preprocessing.StandardScaler
on the columnsx1
andx2
, scaling to zero mean and unit standard deviation. It is in training mode.This feeds into
cluster_model
which usessklearn.cluster.KMeans
with k=6 (and seed=42) to cluster the data. That feeds to aCSvWriter
and to aClusterPlotter
. the plotter creates a scatterplot with title “Results of KMeans(k=6)” and saving toclusters.png
.
You can now run this primrose job with the following command:
primrose run --config config/hello_world_cluster_simple_train.json
Here is the output:
Congratulations. You have now machined-learned in primrose
!
A Machine Learning Job with Model Caching: Classification
In this last example, we will train another Sklearn classifier model. However, here, we cache the trained model so that it can be used against a new dataset at a later stage—i.e., in separate primrose
job—in either predict
or eval
mode.
The dataset we are using is Tom Mitchell’s play tennis example to illustrate decision trees:
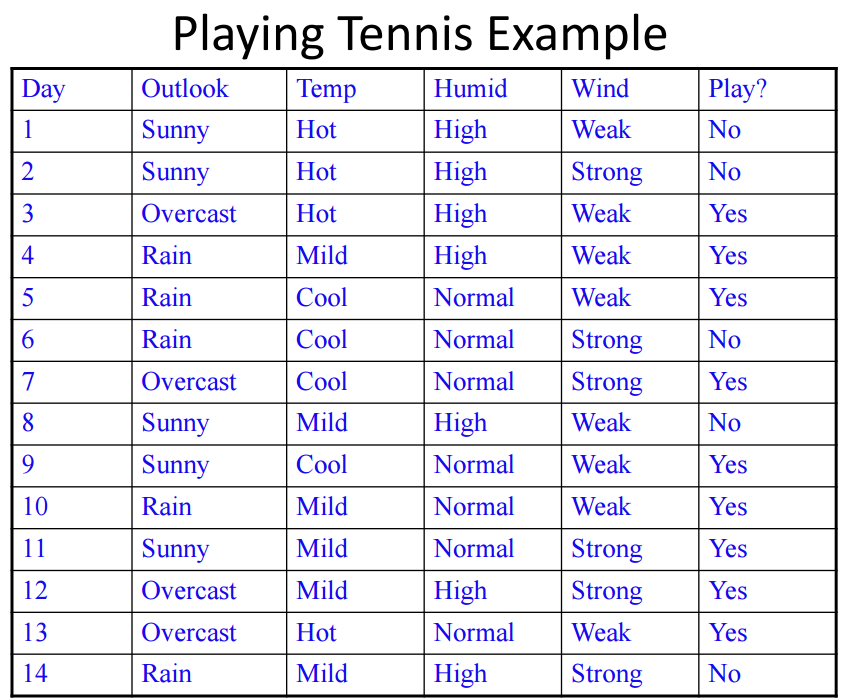
Here, we run two separate primrose
jobs: 1) train and 2) predict. Each job has its own configuration file.
config/hello_world_classifier_train.json
reads the tennis
dataset, does a train test split, runs a Sklearn decision tree model in mode predict
, and writes both the TransformerSequence
(set of transformation performed on the features) and the model
to a local cache using dill
:
...
"encode_and_split": {
"class": "EncodeTrainTestSplit",
"is_training": true,
"target_variable": "play",
"training_fraction": 0.65,
"seed": 42,
"destinations": [
"decision_tree_model",
"write_encoder"
]
}
...
"write_encoder": {
"class": "DillWriter",
"key": "transformer_sequence",
"dir": "cache",
"filename": "hello_world_encoder.dill"
},
"write_model": {
"class": "DillWriter",
"key": "model",
"dir": "cache",
"filename": "hello_world_model.dill"
}
A separate configuration file, config/hello_world_classifier_predict.json
is designed to make predictions using the cached model.
Ideally, we would use a different dataset to make predictions on, but we are using the same tennis dataset here for simplicity.
In this configuration, we read the data and the two cached objects:
"reader_config": {
"read_data": {
"class": "CsvReader",
"filename": "data/tennis.csv",
"destinations": [
"encode_and_split"
]
},
"read_model": {
"class": "DillReader",
"filename": "cache/hello_world_model.dill",
"destinations": [
"decision_tree_model"
]
},
"read_encoder": {
"class": "DillReader",
"filename": "cache/hello_world_encoder.dill",
"destinations": [
"encode_and_split"
]
}
},
run through the model with mode: predict
:
"decision_tree_model": {
"class": "SklearnClassifierModel",
"sklearn_classifier_name": "tree.DecisionTreeClassifier",
"mode": "predict",
"grid_search_scoring": null,
"cv_folds": null,
"destinations": [
"write_output"],
"model_parameters": {}
}
and, finally, write out the predictions
"writer_config": {
"write_output": {
"class": "CsvWriter",
"key": "predictions",
"dir": "cache",
"filename": "hello_world_predictions.csv"
}
}
Try running it yourself. First, run
primrose run --config config/hello_world_classifier_train.json
and inspect the encoder and model files in the cache
folder.
Now run the predict job:
primrose run --config config/hello_world_classifier_predict.json
and find the predictions in the cache
folder.
You are now set up to design realistic primrose
runs in a production setting.
Next
Learn more about primrose
configurations: DAG Configurations